Machine learning is revolutionizing the automobile sector in various ways. Machine learning is driving innovation and transformation in the automobile industry, paving the way for safer, more efficient, and sustainable transportation. As technology continues to advance, the integration of machine learning in vehicles will further accelerate, shaping the future of mobility.
Here are some ways in which machine learning is transforming the automobile industry:
1. Autonomous Driving: Machine learning algorithms are a key component of self-driving cars. These algorithms analyze sensor data from cameras, LiDAR, radar, and other sources to perceive the surrounding environment, detect objects, and make real-time decisions for safe navigation. Machine learning enables autonomous vehicles to learn from data and improve their driving capabilities over time.
2. Advanced Driver Assistance Systems (ADAS): Machine learning is used in ADAS to enhance driver safety and convenience. Algorithms can detect lane departures, recognize traffic signs and signals, predict potential collisions, and assist with features like adaptive cruise control, automatic emergency braking, and blind-spot detection.
3. Predictive Maintenance: Machine learning algorithms analyze vehicle sensor data to predict maintenance needs and prevent breakdowns. By monitoring various parameters such as engine performance, tire wear, and battery health, machine learning models can identify patterns and anomalies, enabling proactive maintenance scheduling and reducing vehicle downtime.
4. Vehicle Diagnostics and Prognostics: Machine learning techniques are employed to diagnose and predict vehicle faults and failures. Algorithms can analyze data from onboard sensors and historical maintenance records to identify potential issues, recommend repairs, and optimize vehicle performance.
5. Customer Experience and Personalization: Machine learning enables automakers to provide personalized experiences to customers. By analyzing customer data, including preferences, driving behavior, and usage patterns, machine learning models can customize in-car features, infotainment systems, and assist with navigation, making the driving experience more enjoyable and tailored to individual needs.
6. Traffic Prediction and Optimization: Machine learning algorithms can analyze historical traffic data, real-time information, and weather conditions to predict traffic patterns and congestion. This information can be used to optimize routes, improve navigation systems, and enable smarter traffic management for efficient and eco-friendly transportation.
7. Vehicle Insurance and Risk Assessment: Machine learning models are used by insurance companies to assess risk factors and determine insurance premiums. By analyzing driver behavior data, including speed, acceleration, and braking patterns, machine learning algorithms can provide accurate risk assessments and enable usage-based insurance policies.
8. Supply Chain and Logistics: Machine learning is applied in supply chain management and logistics to optimize inventory management, route planning, and demand forecasting. Algorithms can analyze historical data, market trends, and customer behavior to optimize logistics operations, reduce costs, and improve delivery efficiency.
9. Manufacturing and Quality Control: Machine learning is used in automotive manufacturing for quality control and defect detection. Algorithms can analyze sensor data, images, and production parameters to identify anomalies, detect defects, and improve manufacturing processes, ensuring high-quality vehicles.
10. Energy Efficiency and Sustainability: Machine learning algorithms can optimize vehicle energy consumption by analyzing driving patterns, road conditions, and battery performance. This helps in improving fuel efficiency, range optimization for electric vehicles, and reducing environmental impact.
11. Voice Recognition and Natural Language Processing: Machine learning algorithms enable voice recognition systems in cars, allowing drivers to interact with their vehicles through voice commands. Natural language processing techniques understand and respond to spoken instructions, enabling hands-free operation and enhancing the overall user experience.
12. Smart Predictive Navigation: Machine learning algorithms can learn from historical data and user preferences to provide smart predictive navigation. By analyzing factors such as time of day, traffic conditions, and personal habits, the navigation system can offer real-time route suggestions, optimize travel times, and provide personalized recommendations for stops along the way.
13. Gesture Recognition: Machine learning enables gesture recognition systems in vehicles, allowing drivers to control various functions with hand gestures. This hands-free interaction enhances safety and convenience, as drivers can adjust settings, answer calls, or control infotainment systems without taking their hands off the steering wheel.
14. Fleet Management and Optimization: Machine learning algorithms assist in fleet management by optimizing routes, managing vehicle assignments, and predicting maintenance needs. Fleet operators can utilize machine learning models to improve operational efficiency, reduce fuel consumption, and enhance overall fleet performance.
15. Smart Parking Solutions: Machine learning is applied to develop smart parking systems that use sensor data and historical patterns to predict parking availability. Drivers can access real-time parking information, locate available spaces, and minimize the time spent searching for parking, reducing traffic congestion and emissions.
16. Cybersecurity and Threat Detection: Machine learning techniques are used to enhance automotive cybersecurity. Algorithms can detect and mitigate potential cyber threats, such as unauthorized access or malware attacks, safeguarding vehicle systems and protecting sensitive data.
17. Vehicle-to-Vehicle Communication: Machine learning facilitates vehicle-to-vehicle (V2V) communication, enabling cars to share real-time data with each other. This data exchange allows vehicles to coordinate movements, share traffic information, and improve safety by detecting potential collision risks.
18. Energy Harvesting and Efficiency: Machine learning algorithms can optimize energy harvesting in electric vehicles by analyzing factors such as driving patterns, terrain, and energy consumption. This enables efficient energy regeneration, improved battery management, and extended range for electric vehicles.
19. Emotion Recognition and Driver Monitoring: Machine learning models can analyze driver behavior and facial expressions to recognize emotions and monitor driver attention and fatigue levels. This technology can help detect drowsiness, distraction, or aggressive driving, promoting safer driving practices and reducing accidents.
20. Connected Car Analytics: Machine learning is utilized to analyze the vast amount of data generated by connected cars. These insights can be leveraged for personalized marketing, vehicle performance monitoring, predictive maintenance, and the development of new services and features tailored to customer needs.
Machine learning continues to push the boundaries of innovation in the automobile industry, transforming the way vehicles are designed, manufactured, operated, and experienced. With ongoing advancements, we can expect further improvements in safety, efficiency, connectivity, and sustainability in the automotive sector.
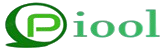
Terimakasih telah membaca di Piool.com, semoga bermanfaat dan lihat juga di situs berkualitas dan paling populer Aopok.com, peluang bisnis online Topbisnisonline.com dan join di komunitas Topoin.com.